SI1922423419: Perbedaan revisi
[revisi tidak terperiksa] | [revisi tidak terperiksa] |
Arbinuriman (bicara | kontrib) (Arbi Nuriman) |
Arbinuriman (bicara | kontrib) (Arbi Nuriman) |
||
Baris 4: | Baris 4: | ||
<div style="font-size: 16pt;font-family: 'times new roman';text-align: center;"> | <div style="font-size: 16pt;font-family: 'times new roman';text-align: center;"> | ||
− | <p style="line-height: 1">''' | + | <p style="line-height: 1">'''OPTIMALISASI SISTEM REKOMENDASI STARTUP'''</p> |
− | <p style="line-height: 1">''' | + | <p style="line-height: 1">'''MENGGUNAKAN METODE RANDOM FOREST'''</p> |
+ | </div> | ||
<div style="font-size: 16pt;font-family: 'times new roman';text-align: center;"> | <div style="font-size: 16pt;font-family: 'times new roman';text-align: center;"> | ||
− | <p style="line-height: 1">''' | + | <p style="line-height: 1">'''SKRIPSI'''</P></div> |
− | <div align="center"><img width="170" height="170" style="margin:0px" src="https:// | + | <div align="center"><img width="170" height="170" style="margin:0px" src="https://drive.pastibisa.app/1706520596_65b77014ba8a8.png"/></div> |
Baris 26: | Baris 27: | ||
{|table align="center" | {|table align="center" | ||
|- | |- | ||
− | |<div style="font-size: 14pt;font-family: 'times new roman';text-align: left">'''NIM '''</div>||<div style="font-size: 14pt;font-family: 'times new roman';text-align: left">''': | + | |<div style="font-size: 14pt;font-family: 'times new roman';text-align: left">'''NIM '''</div>||<div style="font-size: 14pt;font-family: 'times new roman';text-align: left">''': 1922423419'''</div> |
|- | |- | ||
− | |<div style="font-size: 14pt;font-family: 'times new roman';text-align: left">'''NAMA '''</div>||<div style="font-size: 14pt;font-family: 'times new roman';text-align: left">''': | + | |<div style="font-size: 14pt;font-family: 'times new roman';text-align: left">'''NAMA '''</div>||<div style="font-size: 14pt;font-family: 'times new roman';text-align: left">''': Arbi Nuriman'''</div> |
|} | |} | ||
<div style="font-size: 14pt;font-family: 'times new roman';text-align: center;"> | <div style="font-size: 14pt;font-family: 'times new roman';text-align: center;"> | ||
− | <p style="line-height: 1">''' | + | <p style="line-height: 1">'''FAKULTAS SAINS DAN TEKNOLOGI'''</p></div> |
<div style="font-size: 14pt;font-family: 'times new roman';text-align: center;"> | <div style="font-size: 14pt;font-family: 'times new roman';text-align: center;"> | ||
− | <p style="line-height: 1">'''PROGRAM STUDI | + | <p style="line-height: 1">'''PROGRAM STUDI TEKNIK INFORMATIKA'''</p></div> |
<div style="font-size: 14pt;font-family: 'times new roman';text-align: center;"> | <div style="font-size: 14pt;font-family: 'times new roman';text-align: center;"> | ||
− | <p style="line-height: 1">'''KONSENTRASI | + | <p style="line-height: 1">'''KONSENTRASI SOFTWARE ENGINEERING'''</p></div> |
<div style="font-size: 14pt;font-family: 'times new roman';text-align: center;"> | <div style="font-size: 14pt;font-family: 'times new roman';text-align: center;"> | ||
− | <p style="line-height: 1">''' | + | <p style="line-height: 1">'''UNIVERSITAS RAHARJA'''</p></div> |
<div style="font-size: 14pt;font-family: 'times new roman';text-align: center;"> | <div style="font-size: 14pt;font-family: 'times new roman';text-align: center;"> | ||
<p style="line-height: 1">'''TANGERANG'''</p></div> | <p style="line-height: 1">'''TANGERANG'''</p></div> | ||
<div style="font-size: 14pt;font-family: 'times new roman';text-align: center;"> | <div style="font-size: 14pt;font-family: 'times new roman';text-align: center;"> | ||
− | <p style="line-height: 1">'''TA. | + | <p style="line-height: 1">'''TA. 2023/2024'''</p></div> |
Baris 51: | Baris 52: | ||
<div style="font-size: 14pt;font-family: 'times new roman';text-align: center"><p style="line-height: 2">ABSTRAK</p></div> | <div style="font-size: 14pt;font-family: 'times new roman';text-align: center"><p style="line-height: 2">ABSTRAK</p></div> | ||
− | <div style="font-size: 12pt;font-family: 'times new roman';text-align: justify"><p style="line-height: 1"> | + | <div style="font-size: 12pt;font-family: 'times new roman';text-align: justify"><p style="line-height: 1">Penelitian ini dilatarbelakangi oleh Peraturan Pemerintah berdasarkan Undang-Undang No. 11 Tahun 2020 tentang Cipta Kerja, yang memberikan dukungan sumber daya, termasuk fasilitas untuk mendukung digitalisasi. Dalam konteks ini, keberadaan platform Digital Library menjadi relevan mengingat peran teknologi di era digital. Penelitian ini fokus pada penggunaan metode Random Forest untuk meningkatkan efisiensi dan efektivitas sistem rekomendasi pada aplikasi AIMEE, sebuah platform yang mendukung pertumbuhan startupreneur di Indonesia. AIMEE memfasilitasi matchmaking antara startup, model bisnis, sektor industri, dan berbagai provinsi, serta talenta digital, dengan tujuan mewujudkan smart economy. Permasalahan utama yang dihadapi AIMEE adalah ketidaktersediaan fitur matchmaking antara startup dan investor. Penelitian ini bertujuan untuk mengatasi kekurangan ini dengan mengimplementasikan model machine learning, yaitu Random Forest. Pendekatan pemecahan masalah melibatkan langkah-langkah seperti menetapkan tujuan, pengumpulan data startup, analisis data dengan metode Random Forest, visualisasi hasil analisis, dan optimalisasi data startupreneur. Manfaatnya melibatkan efisiensi pengolahan data bisnis, kemudahan pemahaman data, kemampuan menganalisis data dari berbagai sumber, dan melaporkannya dalam bentuk visual bervariasi sebagai panduan dalam pengambilan keputusan bisnis. Penelitian ini memberikan kontribusi terhadap literatur dengan memadukan regulasi pemerintah, kebutuhan industri startup, dan penerapan teknologi machine learning untuk memperbaiki fitur matchmaking dalam platform AIMEE. |
+ | |||
</p></div> | </p></div> | ||
<div style="font-size: 12pt;font-family: 'times new roman';text-align: justify"> | <div style="font-size: 12pt;font-family: 'times new roman';text-align: justify"> | ||
− | <p style="line-height: 1">''Kata Kunci: '' | + | <p style="line-height: 1">''Kata Kunci: ''AIMEE, Random Forest, Machine Learning, Matchmaking, Optimalisasi Data ''</p></div> |
− | + | ||
Baris 64: | Baris 65: | ||
<p style="line-height: 2">''ABSTRACT''</p></div> | <p style="line-height: 2">''ABSTRACT''</p></div> | ||
− | <div style="font-size: 12pt;font-family: 'times new roman';text-align: justify"><p style="line-height: 1">'' | + | <div style="font-size: 12pt;font-family: 'times new roman';text-align: justify"><p style="line-height: 1">''This research is motivated by the Government Regulation based on Law No. 11 of 2020 concerning Job Creation, which provides resource support, including facilities to support digitisation. In this context, the existence of a Digital Library platform is relevant given the role of technology in the digital era. This research focuses on using the Random Forest method to improve the efficiency and effectiveness of the recommendation system on the AIMEE application, a platform that supports the growth of startupreneurs in Indonesia. AIMEE facilitates matchmaking between startups, business models, industry sectors, and various provinces, as well as digital talents, with the aim of realising a smart economy. The main problem faced by AIMEE is the unavailability of matchmaking features between startups and investors. This research aims to address this shortcoming by implementing a machine learning model, namely Random Forest. The problem-solving approach involves steps such as goal setting, startup data collection, data analysis using Random Forest method, visualisation of analysis results, and optimisation of startupreneur data. The benefits involve the efficiency of business data processing, ease of data understanding, ability to analyse data from various sources, and report it in varied visual forms as a guide in business decision-making. This research contributes to the literature by combining government regulations, indus |
+ | ''</p></div> | ||
<div style="font-size: 12pt;font-family: 'times new roman';text-align: justify"> | <div style="font-size: 12pt;font-family: 'times new roman';text-align: justify"> | ||
− | <p style="line-height: 1">''Keywords : '' | + | <p style="line-height: 1">''Keywords : ''AIMEE, Random Forest, Machine Learning, Matching, Data Optimisation''</p></div> |
− | + | ||
− | ''</p></div> | + | |
Baris 76: | Baris 76: | ||
<div style="font-size: 14pt;font-family: 'times new roman';text-align: center"><p style="line-height: 2">DAFTAR PUSTAKA</p></div> | <div style="font-size: 14pt;font-family: 'times new roman';text-align: center"><p style="line-height: 2">DAFTAR PUSTAKA</p></div> | ||
<div style="font-size: 12pt;font-family: 'times new roman';text-align: justify"><p style="line-height: 1"> | <div style="font-size: 12pt;font-family: 'times new roman';text-align: justify"><p style="line-height: 1"> | ||
− | |||
− | [2] | + | [1] Rahardja U, Aini Q, Santoso NPL. Pengintegrasian YII Framework Berbasis API pada Sistem Penilaian Absensi. Sisfotenika. 2018;8(2):140–52. |
+ | |||
+ | [2] Rahardja U, Aini Q, Khoirunisa A. Implementasi Business Intelligence Menggunakan Highchart pada Sistem Penilaian Absensi berbasis YII Framework. CSRID (Computer Sci Res Its Dev Journal). 2017;9(2):115–24. | ||
+ | |||
+ | [3] Fauziah Z, Latifah H, Rahardja U, Lutfiani N, Mardiansyah A. Designing student attendance information systems web-based. Aptisi Trans Technopreneursh. 2021;3(1):23–31. | ||
+ | |||
+ | [4] Lutfiani N, Rahardja U, Khasanah KT. The Development Viewboard As an Information Media at Official Site Asosiation. ATM [Internet]. 2022 Jan. 8 [cited 2023 Aug. 8];6(1):10-8. Available from: https://ijc.ilearning.co/index.php/ATM/article/view/1529 | ||
+ | |||
+ | [5] Fauziah Z, Latifah H, Rahardja U, Lutfiani N, Mardiansyah A. Designing Student Attendance Information Systems Web-Based . att [Internet]. 2021 Feb. 15 [cited 2023 Aug. 8];3(1):23-31. Available from: https://att.aptisi.or.id/index.php/att/article/view/114. | ||
+ | |||
+ | [6] Zhang, L., Liu, Z., Ren, T., Liu, D., Ma, Z., Tong, L., ... & Li, S. (2020). Identification of seed maize fields with high spatial resolution and multiple spectral remote sensing using random forest classifier. Remote Sensing, 12(3), 362. | ||
− | [ | + | [7] Pal, M. (2005). Random forest classifier for remote sensing classification. International journal of remote sensing, 26(1), 217-222. |
− | [ | + | [8]. Paul, A., Mukherjee, D. P., Das, P., Gangopadhyay, A., Chintha, A. R., & Kundu, S. (2018). Improved random forest for classification. IEEE Transactions on Image Processing, 27(8), 4012-4024. |
− | [ | + | [9] Phan, T. N., Kuch, V., & Lehnert, L. W. (2020). Land cover classification using Google Earth Engine and random forest classifier—The role of image composition. Remote Sensing, 12(15), 2411.rest. R news, 2(3), 18-22. |
− | [ | + | [10] Boateng, E. Y., Otoo, J., & Abaye, D. A. (2020). Basic tenets of classification algorithms K-nearest-neighbor, support vector machine, random forest and neural network: a review. Journal of Data Analysis and Information Processing, 8(4), 341-357. |
− | [ | + | [11] Sheykhmousa, M., Mahdianpari, M., Ghanbari, H., Mohammadimanesh, F., Ghamisi, P., & Homayouni, S. (2020). Support vector machine versus random forest for remote sensing image classification: A meta-analysis and systematic review. IEEE Journal of Selected Topics in Applied Earth Observations and Remote Sensing, 13, 6308-6325. |
− | [ | + | [12] Hatwell, J., Gaber, M. M., & Azad, R. M. A. (2020). CHIRPS: Explaining random forest classification. Artificial Intelligence Review, 53, 5747-5788. |
− | [ | + | [13] Zhang, T., Su, J., Xu, Z., Luo, Y., & Li, J. (2021). Sentinel-2 satellite imagery for urban land cover classification by optimized random forest classifier. Applied Sciences, 11(2), 543. |
− | [ | + | [14] Jackins, V., Vimal, S., Kaliappan, M., & Lee, M. Y. (2021). AI-based smart prediction of clinical disease using random forest classifier and Naive Bayes. The Journal of Supercomputing, 77, 5198-5219 |
− | [ | + | [15] Yang, P., Wang, D., Zhao, W. B., Fu, L. H., Du, J. L., & Su, H. (2021). Ensemble of kernel extreme learning machine based random forest classifiers for automatic heartbeat classification. Biomedical Signal Processing and Control, 63, 102138 |
− | [ | + | [16] Zakariazadeh, A. (2022). Smart meter data classification using optimized random forest algorithm. ISA transactions, 126, 361-369 |
− | [ | + | [17] Asadi, S., Roshan, S., & Kattan, M. W. (2021). Random forest swarm optimization-based for heart diseases diagnosis. Journal of biomedical informatics, 115, 103690. |
− | [ | + | [18] Mostafiz, R., Uddin, M. S., Reza, M. M., & Rahman, M. M. (2022). Covid-19 detection in chest X-ray through random forest classifier using a hybridization of deep CNN and DWT optimized features. Journal of King Saud University-Computer and Information Sciences, 34(6), 3226-3235. |
− | [ | + | [19] Rong, G., Alu, S., Li, K., Su, Y., Zhang, J., Zhang, Y., & Li, T. (2020). Rainfall induced landslide susceptibility mapping based on Bayesian optimized random forest and gradient boosting decision tree models—A case study of Shuicheng County, China. Water, 12(11), 3066. |
− | [ | + | [20] Dachi, J. M. A. S., & Sitompul, P. (2023). Analisis Perbandingan Algoritma XGBoost dan Algoritma Random Forest Ensemble Learning pada Klasifikasi Keputusan Kredit. Jurnal Riset Rumpun Matematika dan Ilmu Pengetahuan Alam (JURRIMIPA), 2(2), 87-103. |
− | [ | + | [21] Riza, F. (2021). Analisis dan Prediksi Data Penjualan Menggunakan Machine Learning dengan Pendekatan Ilmu Data. Data Sciences Indonesia (DSI), 1(2), 62-68. |
− | [ | + | [22] Husein, A. M., Harahap, M., & Fernandito, P. (2021). Pendekatan Data Science untuk Menemukan Churn Pelanggan pada Sector Perbankan dengan Machine Learning. Data Sciences Indonesia (DSI), 1(1), 8-13. |
− | [ | + | [23] Nopianti, P. D. (2022). Analisis Data Guna Meningkatkan Optimasi Perusahaan. Journal of Comprehensive Science (JCS), 1(2), 214-221. |
− | [ | + | [24] Yasir, M., & Suraji, R. (2023). PERBANDINGAN METODE KLASIFIKASI NAÏVE BAYES, DECISION TREE, RANDOM FOREST TERHADAP ANALISIS SENTIMEN KENAIKAN BIAYA HAJI 2023 PADA MEDIA SOSIAL YOUTUBE. Jurnal Cahaya Mandalika, 3(2), 180-192. |
Revisi per 9 Februari 2024 09.51
OPTIMALISASI SISTEM REKOMENDASI STARTUP
MENGGUNAKAN METODE RANDOM FOREST
SKRIPSI
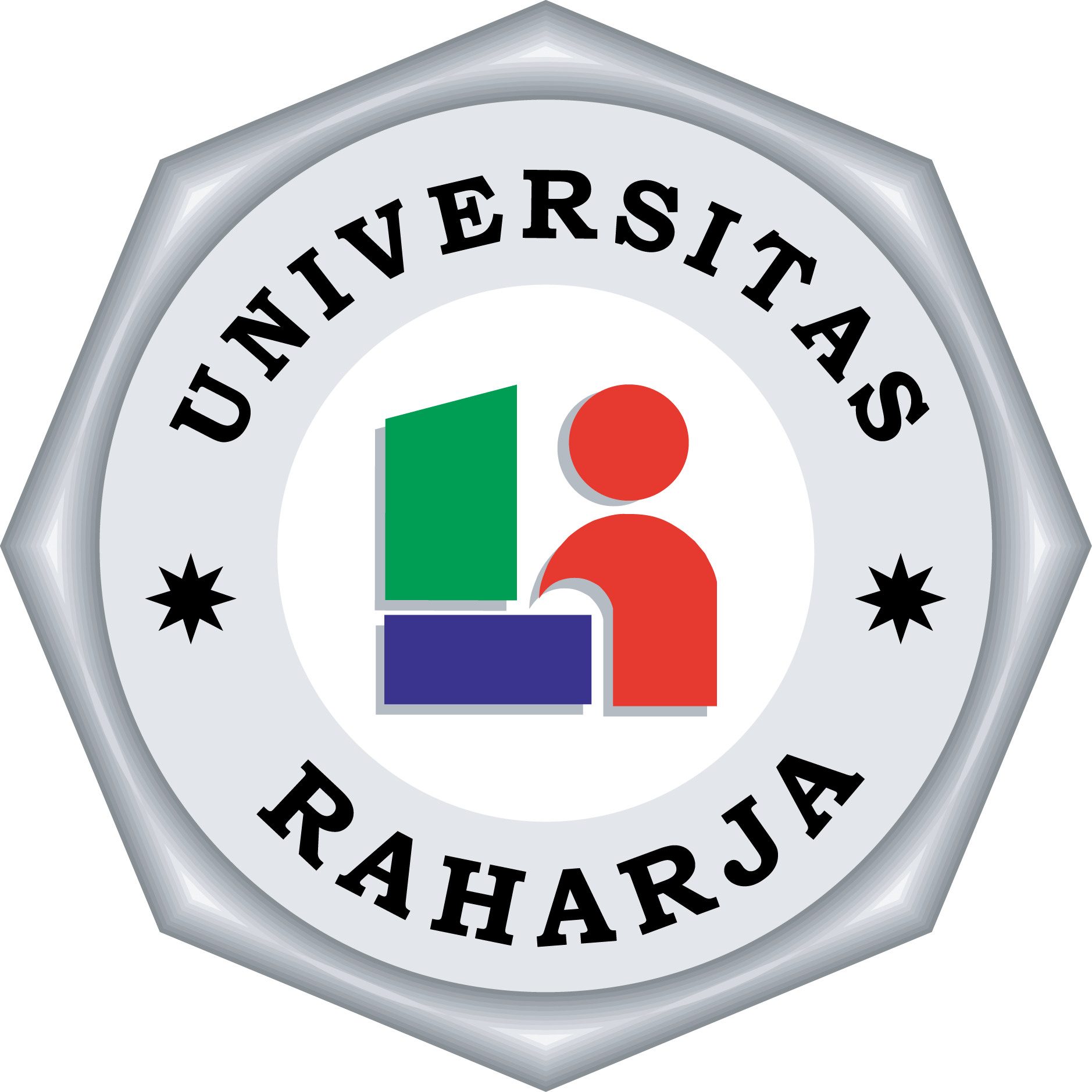
Disusun Oleh :
NIM |
: 1922423419
|
NAMA |
: Arbi Nuriman
|
FAKULTAS SAINS DAN TEKNOLOGI
PROGRAM STUDI TEKNIK INFORMATIKA
KONSENTRASI SOFTWARE ENGINEERING
UNIVERSITAS RAHARJA
TANGERANG
TA. 2023/2024
ABSTRAK
Penelitian ini dilatarbelakangi oleh Peraturan Pemerintah berdasarkan Undang-Undang No. 11 Tahun 2020 tentang Cipta Kerja, yang memberikan dukungan sumber daya, termasuk fasilitas untuk mendukung digitalisasi. Dalam konteks ini, keberadaan platform Digital Library menjadi relevan mengingat peran teknologi di era digital. Penelitian ini fokus pada penggunaan metode Random Forest untuk meningkatkan efisiensi dan efektivitas sistem rekomendasi pada aplikasi AIMEE, sebuah platform yang mendukung pertumbuhan startupreneur di Indonesia. AIMEE memfasilitasi matchmaking antara startup, model bisnis, sektor industri, dan berbagai provinsi, serta talenta digital, dengan tujuan mewujudkan smart economy. Permasalahan utama yang dihadapi AIMEE adalah ketidaktersediaan fitur matchmaking antara startup dan investor. Penelitian ini bertujuan untuk mengatasi kekurangan ini dengan mengimplementasikan model machine learning, yaitu Random Forest. Pendekatan pemecahan masalah melibatkan langkah-langkah seperti menetapkan tujuan, pengumpulan data startup, analisis data dengan metode Random Forest, visualisasi hasil analisis, dan optimalisasi data startupreneur. Manfaatnya melibatkan efisiensi pengolahan data bisnis, kemudahan pemahaman data, kemampuan menganalisis data dari berbagai sumber, dan melaporkannya dalam bentuk visual bervariasi sebagai panduan dalam pengambilan keputusan bisnis. Penelitian ini memberikan kontribusi terhadap literatur dengan memadukan regulasi pemerintah, kebutuhan industri startup, dan penerapan teknologi machine learning untuk memperbaiki fitur matchmaking dalam platform AIMEE.
Kata Kunci: AIMEE, Random Forest, Machine Learning, Matchmaking, Optimalisasi Data
ABSTRACT
This research is motivated by the Government Regulation based on Law No. 11 of 2020 concerning Job Creation, which provides resource support, including facilities to support digitisation. In this context, the existence of a Digital Library platform is relevant given the role of technology in the digital era. This research focuses on using the Random Forest method to improve the efficiency and effectiveness of the recommendation system on the AIMEE application, a platform that supports the growth of startupreneurs in Indonesia. AIMEE facilitates matchmaking between startups, business models, industry sectors, and various provinces, as well as digital talents, with the aim of realising a smart economy. The main problem faced by AIMEE is the unavailability of matchmaking features between startups and investors. This research aims to address this shortcoming by implementing a machine learning model, namely Random Forest. The problem-solving approach involves steps such as goal setting, startup data collection, data analysis using Random Forest method, visualisation of analysis results, and optimisation of startupreneur data. The benefits involve the efficiency of business data processing, ease of data understanding, ability to analyse data from various sources, and report it in varied visual forms as a guide in business decision-making. This research contributes to the literature by combining government regulations, indus
Keywords : AIMEE, Random Forest, Machine Learning, Matching, Data Optimisation
DAFTAR PUSTAKA
[1] Rahardja U, Aini Q, Santoso NPL. Pengintegrasian YII Framework Berbasis API pada Sistem Penilaian Absensi. Sisfotenika. 2018;8(2):140–52.
[2] Rahardja U, Aini Q, Khoirunisa A. Implementasi Business Intelligence Menggunakan Highchart pada Sistem Penilaian Absensi berbasis YII Framework. CSRID (Computer Sci Res Its Dev Journal). 2017;9(2):115–24.
[3] Fauziah Z, Latifah H, Rahardja U, Lutfiani N, Mardiansyah A. Designing student attendance information systems web-based. Aptisi Trans Technopreneursh. 2021;3(1):23–31.
[4] Lutfiani N, Rahardja U, Khasanah KT. The Development Viewboard As an Information Media at Official Site Asosiation. ATM [Internet]. 2022 Jan. 8 [cited 2023 Aug. 8];6(1):10-8. Available from: https://ijc.ilearning.co/index.php/ATM/article/view/1529
[5] Fauziah Z, Latifah H, Rahardja U, Lutfiani N, Mardiansyah A. Designing Student Attendance Information Systems Web-Based . att [Internet]. 2021 Feb. 15 [cited 2023 Aug. 8];3(1):23-31. Available from: https://att.aptisi.or.id/index.php/att/article/view/114.
[6] Zhang, L., Liu, Z., Ren, T., Liu, D., Ma, Z., Tong, L., ... & Li, S. (2020). Identification of seed maize fields with high spatial resolution and multiple spectral remote sensing using random forest classifier. Remote Sensing, 12(3), 362.
[7] Pal, M. (2005). Random forest classifier for remote sensing classification. International journal of remote sensing, 26(1), 217-222.
[8]. Paul, A., Mukherjee, D. P., Das, P., Gangopadhyay, A., Chintha, A. R., & Kundu, S. (2018). Improved random forest for classification. IEEE Transactions on Image Processing, 27(8), 4012-4024.
[9] Phan, T. N., Kuch, V., & Lehnert, L. W. (2020). Land cover classification using Google Earth Engine and random forest classifier—The role of image composition. Remote Sensing, 12(15), 2411.rest. R news, 2(3), 18-22.
[10] Boateng, E. Y., Otoo, J., & Abaye, D. A. (2020). Basic tenets of classification algorithms K-nearest-neighbor, support vector machine, random forest and neural network: a review. Journal of Data Analysis and Information Processing, 8(4), 341-357.
[11] Sheykhmousa, M., Mahdianpari, M., Ghanbari, H., Mohammadimanesh, F., Ghamisi, P., & Homayouni, S. (2020). Support vector machine versus random forest for remote sensing image classification: A meta-analysis and systematic review. IEEE Journal of Selected Topics in Applied Earth Observations and Remote Sensing, 13, 6308-6325.
[12] Hatwell, J., Gaber, M. M., & Azad, R. M. A. (2020). CHIRPS: Explaining random forest classification. Artificial Intelligence Review, 53, 5747-5788.
[13] Zhang, T., Su, J., Xu, Z., Luo, Y., & Li, J. (2021). Sentinel-2 satellite imagery for urban land cover classification by optimized random forest classifier. Applied Sciences, 11(2), 543.
[14] Jackins, V., Vimal, S., Kaliappan, M., & Lee, M. Y. (2021). AI-based smart prediction of clinical disease using random forest classifier and Naive Bayes. The Journal of Supercomputing, 77, 5198-5219
[15] Yang, P., Wang, D., Zhao, W. B., Fu, L. H., Du, J. L., & Su, H. (2021). Ensemble of kernel extreme learning machine based random forest classifiers for automatic heartbeat classification. Biomedical Signal Processing and Control, 63, 102138
[16] Zakariazadeh, A. (2022). Smart meter data classification using optimized random forest algorithm. ISA transactions, 126, 361-369
[17] Asadi, S., Roshan, S., & Kattan, M. W. (2021). Random forest swarm optimization-based for heart diseases diagnosis. Journal of biomedical informatics, 115, 103690.
[18] Mostafiz, R., Uddin, M. S., Reza, M. M., & Rahman, M. M. (2022). Covid-19 detection in chest X-ray through random forest classifier using a hybridization of deep CNN and DWT optimized features. Journal of King Saud University-Computer and Information Sciences, 34(6), 3226-3235.
[19] Rong, G., Alu, S., Li, K., Su, Y., Zhang, J., Zhang, Y., & Li, T. (2020). Rainfall induced landslide susceptibility mapping based on Bayesian optimized random forest and gradient boosting decision tree models—A case study of Shuicheng County, China. Water, 12(11), 3066.
[20] Dachi, J. M. A. S., & Sitompul, P. (2023). Analisis Perbandingan Algoritma XGBoost dan Algoritma Random Forest Ensemble Learning pada Klasifikasi Keputusan Kredit. Jurnal Riset Rumpun Matematika dan Ilmu Pengetahuan Alam (JURRIMIPA), 2(2), 87-103.
[21] Riza, F. (2021). Analisis dan Prediksi Data Penjualan Menggunakan Machine Learning dengan Pendekatan Ilmu Data. Data Sciences Indonesia (DSI), 1(2), 62-68.
[22] Husein, A. M., Harahap, M., & Fernandito, P. (2021). Pendekatan Data Science untuk Menemukan Churn Pelanggan pada Sector Perbankan dengan Machine Learning. Data Sciences Indonesia (DSI), 1(1), 8-13.
[23] Nopianti, P. D. (2022). Analisis Data Guna Meningkatkan Optimasi Perusahaan. Journal of Comprehensive Science (JCS), 1(2), 214-221.
[24] Yasir, M., & Suraji, R. (2023). PERBANDINGAN METODE KLASIFIKASI NAÏVE BAYES, DECISION TREE, RANDOM FOREST TERHADAP ANALISIS SENTIMEN KENAIKAN BIAYA HAJI 2023 PADA MEDIA SOSIAL YOUTUBE. Jurnal Cahaya Mandalika, 3(2), 180-192.